Your AI Sucks: Part 1
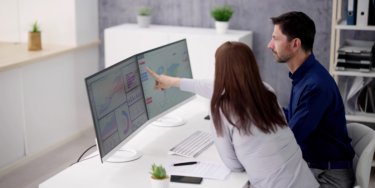
AI, Eh? – Why Businesses Are Adopting AI
Artificial Intelligence is nothing new – as early as 1951, ‘intelligent’ computer programs were being built, and there have been advancements in the field that from time to time have made it into the public eye.
AI has recently become ubiquitous in all walks of life – from online assistants and social media bots to business meetings and post-work pint banter. AI has been brought to the masses – and although the value can seem questionable at times, and the media are churning up scare stories about job security, it’s going nowhere.
This widespread exposure to AI in our day-to-day lives has also had an impact on the way that AI is being adopted by organisations to support and augment their workforce. Xpedition has just completed its roll-out of Copilot for Microsoft 365, which has seen swift adoption across all teams within the business. And we’re not alone—it’s estimated that 35% of businesses are using AI in some way, shape, or form.
And when you look at the numbers, it’s not surprising…
This race to get on the AI rocket ship is being fuelled by reports from analysts who are talking about the potential to automate up to 70% of business activities and reduce costs by 44%. The UK AI market offers huge potential for vendors, with a value of over £16 billion today set to grow to a staggering £800 billion by 2035.
Here Be Monsters! – AI Risks and Challenges
It’s not all smooth sailing, though. There have been numerous reports of where AI has got it wrong—sweary chat-bots, algorithms with racial bias, and financial scams powered by deepfakes. There are also very real concerns about privacy, security, and transparency in the AI that companies are asking their customers to interact with.
So how are organisations supposed to make the most of this technology when the risks of getting it wrong can have such an impact?
Trust.
Before companies can roll out AI at scale, they need to be sure that they can trust the machine. The machine is only as good as the information it is fed. Which is why organisations need to build a solid data foundation. A data foundation that will provide…
Trusted Data.
Garbage in = garbage out
“Pray, Mr. Babbage, if you put into the machine wrong figures, will the right answers come out?” … I am not able rightly to apprehend the kind of confusion of ideas that could provoke such a question.
— Charles Babbage, Passages from the Life of a Philosopher
The amount of trust that a user can put in a system is directly proportionate to the quality of the output. We know from the age-old adage that the quality of the output is directly dependent on the quality of the input data.
Your AI sucks because your data quality sucks—and there are several ways that this can manifest…
- Quality of Training Data: An AI model’s accuracy is driven by its training data. If the data is of limited scope, poorly labelled, or not reflective of real-world scenarios, the model won’t perform as expected when deployed.
- Bias and Fairness: Data can carry biases, which AI systems can then amplify. It’s essential to identify and mitigate these biases to prevent undesirable, discriminatory outcomes.
- Error Propagation: Errors in input data can lead to incorrect AI outputs, affecting decisions and actions based on those outputs.
- Data Integrity and Cleaning: Ensuring the accuracy and consistency of data throughout its lifecycle is crucial for the reliability of AI systems.
In short, for AI to be effective (and therefore trusted), you must ensure that the data used for developing, training, and operating AI models is of high quality, representative, and free from biases.
That’s why, in Part 2, we’ll dive into how to establish a robust data quality strategy to ensure your AI initiatives are both reliable and trustworthy.