How AI Can Enhance Financial Services
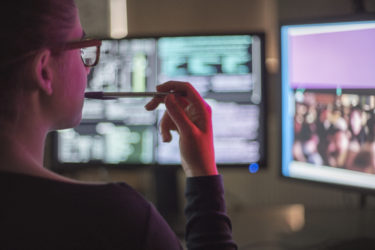
No matter where you look, artificial intelligence (AI) appears to be the future. It has already transformed enterprise and continues to evolve at high speed. Companies across numerous industries are prioritising AI as a key part of innovation, and this is no less true for financial services.
For consumer protection, investment performance, fraud detection, and customer experience, AI offers both an exciting set of capabilities and challenges. Here’s our guide on the uses of AI in finance, and how it is transforming the industry.
AI Explained
Despite the unprecedented advances and interest in AI in recent years, its development stretches back decades. The term was first coined 1956. Under the leadership of John McCarthy, researchers in language simulation, complexity theory, neuron nets, and the learning machines convened in what would be known as the first conference on ‘artificial intelligence’.
Given the extraordinary leaps in the capacity of electronics and computers, their ambition was to investigate ‘how to make machines use language, form abstractions and concepts, solve kinds of problems now reserved for humans, and improve themselves’.
Despite their foresight, the advancements in AI we’ve since seen would no doubt still surprise them. Today, AI is no longer the domain of specialists. In fact, it would be easier to keep track of the sectors where AI is not being at least tested, let alone implemented.
AI in Financial Services
The rise of financial technologies (fintechs), is transforming the finance industry. Established banks are grappling with their own legacy systems while smaller and more agile innovators emerge on the market. Even without sector competition, when it comes to digital transformation, financial services lag behind other consumer-centric industries. Investing in AI may well be the key to solving this, but how, exactly?
In the words of the Alan Turing Institute, ‘the adoption of AI in financial services is underpinned by three distinct elements of innovation: machine learning (ML), non-traditional data, and automation’. Unsurprisingly, the most widespread and influential AI-enabled tools in financial services are machine learning, alternative data, and automation.
Machine Learning
ML describes how computer systems can perform tasks based on their ‘learning’ process drawn from data. Unlike older software that encodes explicit rules and logical statements, ML systems can adapt with no additional instruction input from humans. Instead, they use algorithms and statistical models to recognise and analyse data patterns.
As we explore later on, machine learning in financial services is now considered essential for risk assessment, fraud detection and other vital measures.
Alternative Data
In financial services, alternative or ‘non-traditional’ data is any information that can support investments that goes beyond company filings or other traditional sources.
AI can process both ‘structured’ data for credit risk profiling, and ‘unstructured’ data, importing information such as satellite imagery, news reports to make stock or commodity predictions and make more informed decisions around asset management.
The Benefits of AI in Financial Services
AI is not new to the financial services industry. As with numerous other industries, financial services have adopted chatbots as a customer service solution.
The potential of AI for financial services goes far beyond this, however, and the rate of innovation is accelerating. Here are just a few examples of where AI is transforming financial services for the better:
Fraud Detection
As computer technology has become more sophisticated, so has cybercrime and other fraudulent activities. Banks and financial institutions follow strict regulatory frameworks, but AI models allow them to better fight fraud through (among other methods) advanced data collection, identifying abnormalities and assisting investigators through iterative learning.
ML tools, for example, have been increasingly adopted to enhance decision intelligence, informed by real-time data. Since ML is teachable and can adapt to new learnings, it can automate processes and mitigate problems before they occur, as well as making predictions based on historical and external data.
Organisations can also use generative AI, a subset of ML, to explore potential fraud scenarios through advanced simulation modelling. By using fake data to train machine learning models, they can recognise patterns and rehearse decision-making in difficult and complex fraud situations.
Customer Relationship Management
The value of personalisation is recognised by countless industries, and AI and data science have been instrumental in creating memorable, tailored customer experiences. A growing number of institutions are integrating AI into their customer relationship management (CRM) systems.
Digitisation has meant that customer needs are constantly evolving. To keep up, financial institutions can use algorithms for the intelligent analysis of untold amounts of data, such as transactions, demographics and customer behaviour, to compile customer profiles for targeted, personalised marketing. Beyond chatbots, AI’s rapid access to additional information resources also helps organisations improve in-person customer guidance.
Credit Risk Assessment
The vast majority of AI adoptions in finance are in credit risk analysis. AI algorithms are used to calculate the probability of customer loan defaults, and even the severity of losses. However, it remains the case that many banks’ legacy IT systems are limited to those rule-based AI systems that still require a great deal of human input.
A report from McKinsey suggests that banks need to prioritise machine learning tools across the customer life-cycle, including credit decision-making. In fact, those ‘AI-first banks’ can streamline lending journeys with real-time analysis of data to help a range of business models execute quicker credit decisions.
Bespoke apps like FS Xcelerator help firms adhere to Know Your Client (KYC) checks during the onboarding process, preventing the oversight of critical client information.
The Challenges of AI in Financial Services
As with its application in any industry, AI opens the door to concerns around risk and responsibility. The actual and potential innovations it provides also carry complexities in important areas that should not be overlooked, such as:
Data Governance
AI algorithms need consistent access to vast amounts of data pulled by banks and other institutions in order to properly function. With this access to sensitive financial data and personally-identifiable information (PII) comes the increased risk to customers in the event of a security breach. As the potential for AI to extract data grows, companies must pour additional effort and resources into safeguarding customer data.
A clear data governance framework should outline the institutional policies and procedures for data management. This should be regularly updated to account for developments in AI-enabled tools that harness all types of information across an organisation.
Regulatory Compliance
As the prevalence of AI in financial services grows, it is equally important to ensure systems comply with the strict regulatory framework that banks and other institutions operate within. AI systems can be opaque and complex, which creates a problem when assessing how much power to give bots and other tools that lack human oversight.
While it can play a valuable role in screening and gathering information on customers, AI should not be thought of as a way to completely replace human decision-making in credit approvals. The founder of Black Women in Artificial Intelligence, Angle Bush, noted the risk of using historical data to train algorithms in AI systems: ““This can result in automatic loan denials for individuals from marginalised communities, reinforcing racial or gender disparities”.
Cybersecurity
Financial institutions have long been the target of bad actors, and while AI can be used defensively as part of a company’s security strategy, offensive AI practices now pose a greater threat to an industry dealing with sensitive information and large sums of money.
Fraudsters can use generative AI to enhance efforts to steal sensitive information and money. Phishing is a common tool that many internet users will have encountered, but more sophisticated AI-enabled attacks include deepfakes, password-cracking algorithms, and supply chain attacks, in addition to other potential threats targeting financial institutions.
Conclusion
The digital transformation of financial services digital transformation is well underway, and the use of AI to leverage data is the prize asset of any competitive firm.
Xpedition’s targeted FS Xcelerator suite of apps and Metis FS can help firms accelerate the transition to AI. Our in-depth expertise of regulations and AI-powered tools help businesses compete and thrive in this exciting, challenging new landscape.